The Transformative Power of Labeled Image Datasets in Modern Business
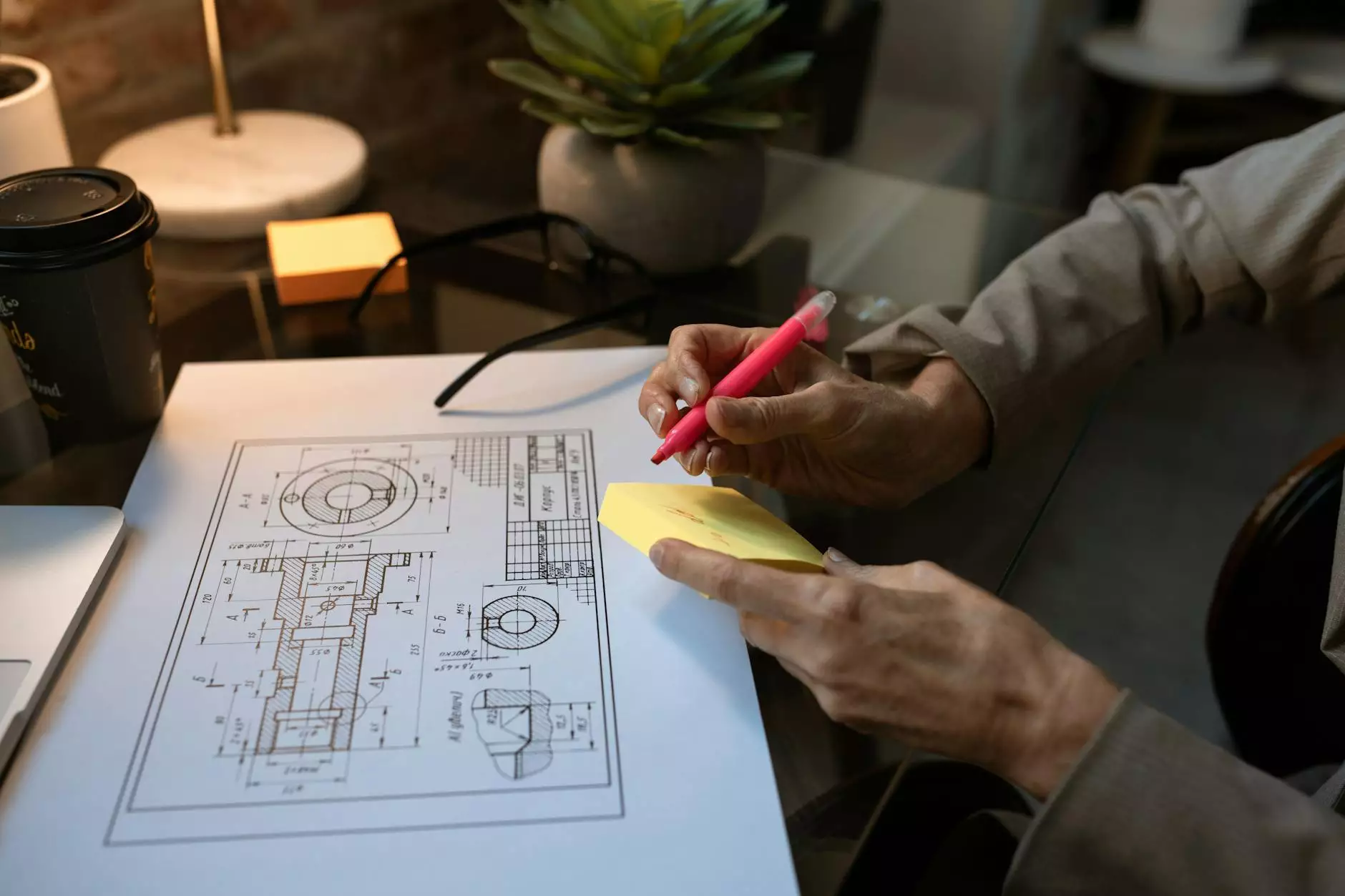
In today's digital age, where data reigns supreme, the significance of labeled image datasets cannot be overstated. These datasets are not just collections of images; they are the cornerstone of machine learning models, providing the necessary training data for AI applications across various industries. At Keylabs.ai, we specialize in delivering robust data annotation tools and platforms that facilitate the creation of these essential datasets, enabling businesses to harness the full potential of AI.
Understanding Labeled Image Datasets
Labeled image datasets consist of images that are annotated with specific tags or labels, allowing algorithms to learn from these instances to perform various tasks efficiently. For example, in the realm of computer vision, these datasets are critical for training models that can recognize objects, interpret scenes, and even predict outcomes based on visual inputs.
The Structure of a Labeled Image Dataset
Typically, a labeled image dataset includes:
- Images: The visual data that serves as the primary input for models.
- Labels: Annotations or classifications associated with each image, detailing what the model should learn from it.
- Metadata: Additional information about the images, such as resolution, capture date, and context.
Importance of Data Annotation in Creating Labeled Image Datasets
Data annotation is the process that transforms raw data into a structured format that machines can understand. When it comes to labeled image datasets, data annotation takes on a crucial role, as it governs the accuracy and reliability of the AI models trained on this data. Here are some key points regarding the importance of data annotation:
1. Accuracy in AI Training
Properly annotated data ensures that AI models learn the right features from images. For instance, when training a model for image classification, precise labeling helps the system distinguish between different classes effectively.
2. Scalability and Efficiency
As businesses scale and more data is generated, the demand for high-quality labeled datasets increases. Utilizing advanced data annotation platforms makes it possible to manage this demand efficiently, allowing for quick adaptations and updates to datasets as necessary.
3. Enhanced Model Performance
Models trained on diverse and well-labeled datasets perform better. This means businesses can leverage technology to its fullest, providing improved services and products to their customers.
Utilizing Keylabs.ai for Data Annotation
At Keylabs.ai, we empower businesses with advanced tools and platforms specifically designed for data annotation. Here’s how we stand out:
Robust Data Annotation Tools
Our suite of data annotation tools streamlines the process, ensuring that teams can efficiently label images while maintaining high levels of accuracy. With features like:
- Automated labeling assistance: Use AI-driven algorithms to make the annotation process quicker and more efficient.
- Customizable workflows: Tailor your annotation process to fit specific project requirements and goals.
- Collaboration features: Facilitate teamwork with real-time collaboration tools that keep everyone on the same page.
Comprehensive Data Annotation Platform
Our platform is designed to handle vast amounts of data. It offers:
- End-to-end solutions: From uploading datasets to receiving final annotations, our platform supports the entire lifecycle.
- Quality assurance: Built-in validation processes ensure that every labeled image dataset meets the highest standards of quality.
- Integration capabilities: Easily connect with existing workflows or build custom integrations with existing systems.
Applications of Labeled Image Datasets Across Industries
The utility of labeled image datasets spans across various sectors, each harnessing the power of annotated data differently. Here are some applications that highlight this versatility:
1. Healthcare
In the medical field, labeled image datasets are pivotal in training models for diagnostics, predictive analytics, and even treatment planning. By accurately labeling medical images, AI can assist physicians in identifying diseases, leading to better patient outcomes.
2. Automotive
Autonomous vehicles rely heavily on labeled image datasets for object detection and scene understanding. With precise annotations, these vehicles can navigate complex environments safely and efficiently, ensuring passenger safety.
3. Retail and E-commerce
In retail, companies utilize labeled image datasets for various purposes, including personalized recommendations and inventory management. By analyzing customer behavior through images, businesses can optimize their marketing strategies.
4. Agriculture
Farmers are increasingly using AI-driven tools that rely on labeled image datasets to monitor crop health, optimize yields, and protect resources. Through remote sensing and image analysis, they can make informed decisions that significantly impact productivity.
Challenges in Creating Labeled Image Datasets
Despite the advantages, creating quality labeled image datasets often comes with challenges that businesses must navigate:
1. Scale and Complexity
As datasets grow, the complexity of annotating each image increases exponentially. Managing large volumes of diverse data can become overwhelming without the right tools and processes in place.
2. Consistency and Quality Control
Maintaining high-quality annotations across teams can be difficult. Inconsistent labeling can lead to model inaccuracies, which can be detrimental in high-stakes environments such as healthcare or automotive safety.
3. Cost and Resource Management
The annotation process can be resource-intensive, requiring significant time, effort, and financial investment. Businesses must evaluate the cost-benefit ratio of their data annotation strategies to ensure sustainable growth.
Strategies for Overcoming Data Annotation Challenges
To navigate the challenges associated with labeled image datasets, businesses can adopt several effective strategies:
1. Leverage Advanced Technology
Utilize AI-assisted annotation tools that can automate parts of the labeling process. These tools not only save time but also enhance overall accuracy by providing annotations that can be refined by human experts.
2. Implement Strong Quality Assurance Protocols
Establish thorough quality control measures to review and validate annotations regularly. This practice helps catch errors early and ensures that the datasets remain reliable.
3. Invest in Training and Resources
Equipping your annotation team with the necessary training and resources is vital. A skilled team is more likely to produce consistent and high-quality annotations that contribute positively to model performance.
Future Trends in Labeled Image Datasets
The landscape of labeled image datasets is continuously evolving. Here are some future trends to watch:
1. Increased Use of Synthetic Data
As the demand for diverse datasets grows, synthetic data generation is becoming more popular. This strategy can augment real datasets, enabling models to learn from scenarios that may be rare or difficult to capture in the real world.
2. More Real-time Annotation Capabilities
Advancements in technology are paving the way for real-time data annotation, where images can be labeled on-the-fly during data capture. This capability will revolutionize industries where timeliness is critical.
3. Enhanced Collaboration Techniques
The future may see more collaborative platforms that integrate techniques from various fields, including crowdsourcing, to improve the annotation process and quality of datasets.
Conclusion: Embracing the Future of Labeled Image Datasets with Keylabs.ai
The journey towards leveraging labeled image datasets for AI-driven innovations is not just a technological shift; it’s a strategic imperative for businesses across sectors. With the right data annotation tools and a reliable data annotation platform, such as those offered by Keylabs.ai, companies can stay ahead of the curve, ensuring that they remain competitive in an increasingly data-driven world.
As we move forward, embracing the power of labeled image datasets will be key to unlocking new possibilities in AI and beyond. It's time to invest in data annotation and build the future of intelligent systems.