The Essential Role of Video Labeling Tool Machine Learning in Data Annotation
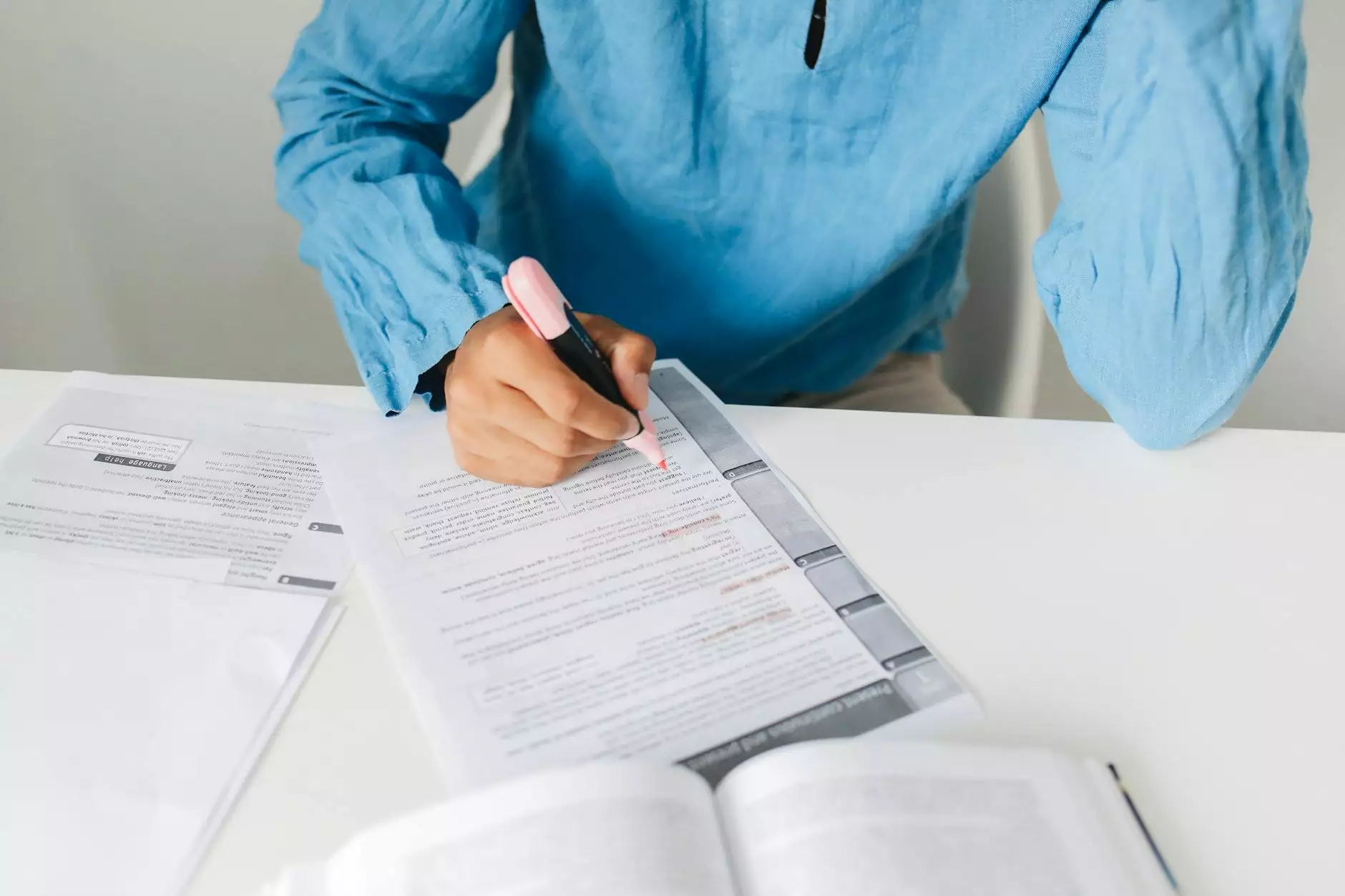
In the constantly evolving world of technology and data, businesses are continuously searching for innovative solutions to improve their workflow and maximize productivity. Among the plethora of tools available, the video labeling tool machine learning has emerged as a crucial element for organizations that rely heavily on data annotation. In this comprehensive article, we will explore the importance of video labeling tools, how machine learning enhances these tools, and the impact they have on businesses today. Join us as we delve into the world of data annotation at Keylabs.ai.
Understanding Video Labeling Tool Machine Learning
At its core, a video labeling tool machine learning is designed to annotate video content with precision and efficiency. This process involves identifying, classifying, and tagging different elements within a video—such as objects, actions, and events—making it a pivotal utility in various applications ranging from autonomous driving to sports analytics.
How Does Machine Learning Enhance Video Labeling?
Machine learning (ML) plays a vital role in optimizing the effectiveness of video labeling tools. By leveraging algorithms that learn from data, these tools can offer superior accuracy and productivity. Here are some of the ways machine learning enhances video labeling:
- Automated Recognition: ML algorithms can automatically identify and categorize objects within a video frame, significantly reducing the time required for manual labeling.
- Improved Accuracy: With continuous learning from new data, machine learning models evolve and improve over time, ensuring higher consistency and accuracy in video annotation.
- Speed Advancements: Machine learning accelerates the annotation process, enabling organizations to annotate large volumes of video data quickly and efficiently.
- Real-time Annotation: Certain advanced ML tools offer real-time video processing and annotation, allowing immediate usage of data in various applications.
The Business Benefits of Video Labeling Tools
Implementing a video labeling tool powered by machine learning can provide numerous advantages for businesses across different sectors. Here are some key benefits:
1. Enhanced Productivity
By automating the video labeling process, businesses can redirect their efforts from tedious, manual tasks to more strategic initiatives. This enhancement in productivity translates to faster project turnaround times and the ability to tackle more projects simultaneously.
2. Cost Efficiency
Investing in a video labeling tool machine learning can ultimately lead to significant cost savings. The reduction in time spent on annotation directly correlates to lower labor costs. Additionally, higher accuracy reduces costly errors that could arise from manual labeling.
3. Scalability
As businesses grow and the amount of video data increases, the need for efficient data annotation becomes even more pressing. Machine learning-enabled tools can scale easily, handling increased volumes of data without compromising quality.
4. Competitive Advantage
In today’s data-driven economy, being able to analyze and utilize video data effectively can give businesses a significant competitive edge. Companies that adopt advanced video labeling tools can leverage insights from their data faster than competitors, allowing for better decision-making and innovative business strategies.
Applications of Video Labeling in Business
The applications for video labeling tools are vast and varied. Here are some prominent use cases:
- Autonomous Vehicles: In the automotive industry, video labeling is crucial for training self-driving cars to recognize pedestrians, traffic signs, and road conditions.
- Healthcare: Medical imaging and diagnostic processes benefit from annotated video data that helps in identifying anomalies and tracking patient progress.
- Sports Analytics: Coaches and analysts utilize annotated game footage to gain insights into player performance, strategies, and game dynamics.
- Surveillance: Security systems use video labeling to detect and identify unusual activities in real-time.
How to Choose the Right Video Labeling Tool
With a plethora of options available in the market, selecting the right video labeling tool powered by machine learning requires careful consideration. Below are important factors to keep in mind:
1. Features and Capabilities
Evaluate the features offered by the tool. Look for capabilities such as real-time annotation, object tracking, and easy export options for integration with other systems.
2. User-Friendliness
The tool should provide an intuitive user interface that minimizes the learning curve for your team. A user-friendly tool maximizes adoption rates and ensures that your organization can leverage its full potential effectively.
3. Scalability Options
Ensure that the tool can handle your current needs while offering scalability options for future growth. As your volume of video data increases, your tool should adapt without performance degradation.
4. Support and Community
Look for tools backed by strong support from developers and an active user community. Good customer support can assist with technical issues and enhance your overall experience with the tool.
5. Pricing Model
Different tools come with varying pricing structures. Explore both subscription and one-time purchase options, ensuring that you choose a model that fits your budget while providing good value for the features offered.
The Future of Video Labeling Tools
The future of video labeling tool machine learning looks promising, with advancements in artificial intelligence and machine learning paving the way for even more sophisticated tools. Here are some trends to watch:
- AI Integration: As AI continues to evolve, we can expect future labeling tools to offer even more advanced features, including predictive analysis and enhanced automation.
- Natural Language Processing: Integration with NLP technologies will allow users to interact with video labeling systems using natural language, making these tools even more accessible.
- Greater Focus on Ethics: As machine learning tools develop, ethical considerations surrounding data privacy and bias will become increasingly important. Companies will need to prioritize these aspects in their video labeling efforts.
Case Studies of Successful Implementations
To illustrate the tangible benefits of employing a video labeling tool machine learning, here are a few notable case studies:
Case Study 1: Automotive Sector
A leading automotive company integrated a video labeling tool into their development of autonomous vehicles, significantly reducing the time required for data annotation from weeks to just days. By harnessing machine learning, they boosted accuracy in object detection, which directly improved their vehicle safety features.
Case Study 2: Healthcare Sector
A prominent hospital network utilized video labeling in their telehealth services, annotating patient interactions to enhance diagnostic accuracy. The tool provided healthcare professionals with insights into patient engagement and outcomes, ultimately leading to improved care strategies.
Conclusion
In conclusion, the adoption of a video labeling tool machine learning can dramatically enhance data annotation processes across various industries. The ability to improve accuracy, speed, and ultimately, business outcomes is invaluable. As organizations continue to pursue data-driven decision-making, investing in sophisticated video labeling solutions will undoubtedly become a standard for success.
Embrace the future of video data with machine learning capabilities and propel your business towards greater efficiency and effectiveness. Explore more at Keylabs.ai to discover how we can help you implement the best solutions for your data annotation needs.